The Impact of AI on Climate Change
As artificial intelligence (AI) becomes increasingly ubiquitous in business and governance, its substantial environmental impact — from significant increases in energy and water usage to heightened carbon emissions — cannot be ignored. By 2030, AI’s power demand is expected to rise by 160%. However, adopting more sustainable practices, such as utilizing foundation models, optimizing data processing locations, investing in energy-efficient processors, and leveraging open-source collaborations, can help mitigate these effects. These strategies not only reduce AI’s environmental footprint but also enhance operational efficiency and cost-effectiveness, balancing innovation with sustainability.
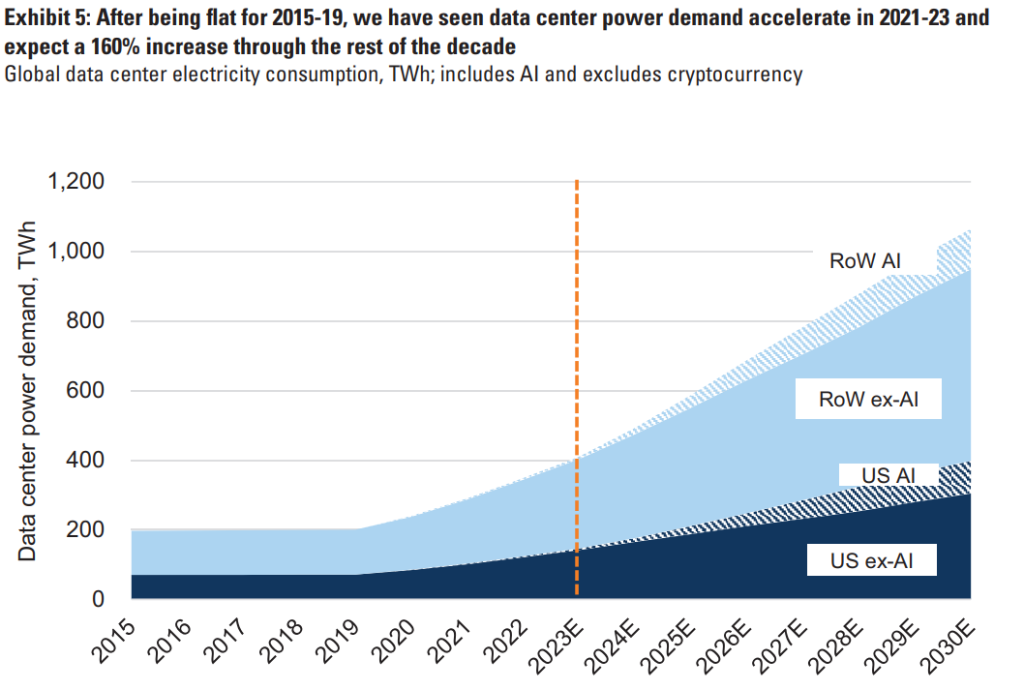
By 2026, computing power dedicated to training AI is expected to increase tenfold. As more power is expended, more resources are needed. Consequently, there have been exponential increases in energy and, perhaps more unexpectedly, water consumption. Some estimates even show that running a large AI model generates more emissions over its lifetime than the average car. A recent report from Goldman Sachs found that by 2030, there will be a 160% increase in demand for power propelled by AI applications.
Sustainable Practices for AI Deployment
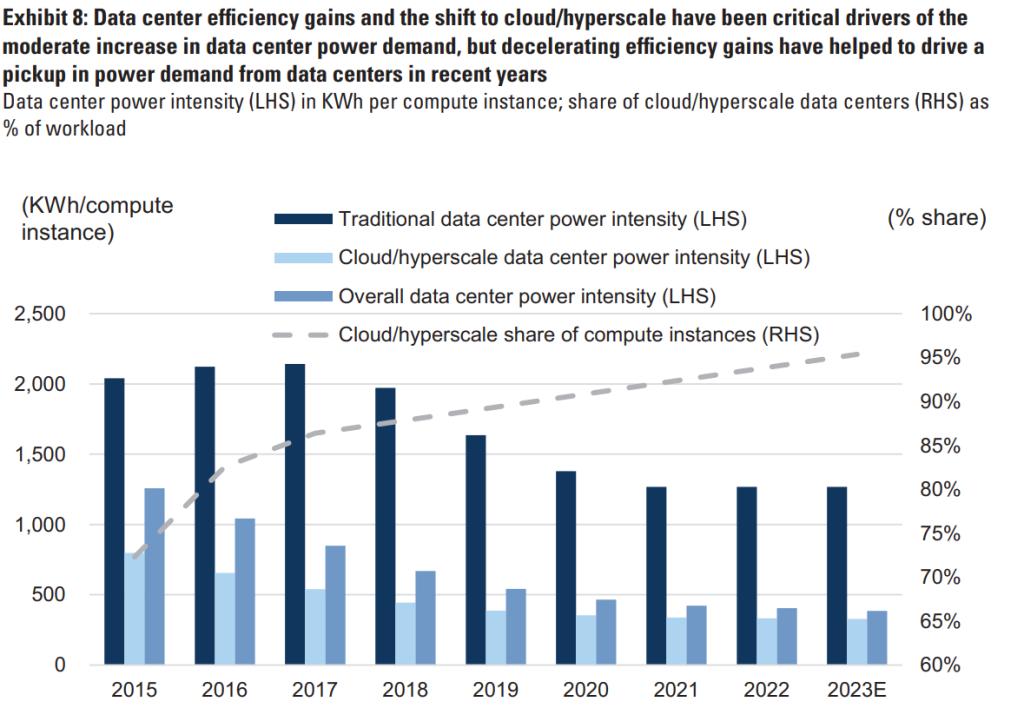
- Make Smart Choices About AI Models
An AI model has three phases — training, tuning, and inferencing — and there are opportunities to be more sustainable at every phase. Business leaders should consider choosing a foundation model rather than creating and training code from scratch. Compared to creating a new model, foundation models can be custom-tuned for specific purposes in a fraction of the time, with a fraction of the data, and a fraction of the energy costs.
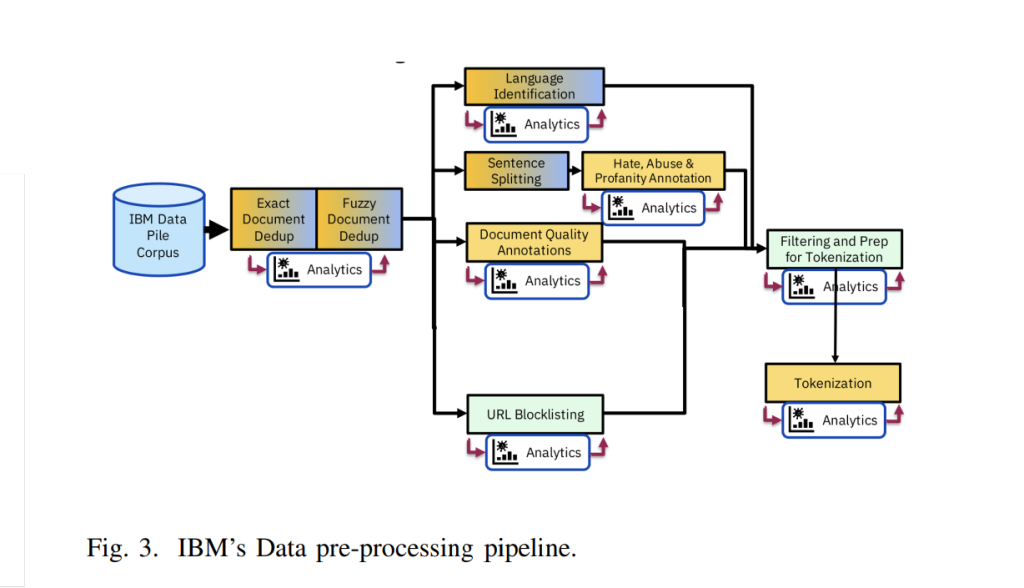
Choosing the right size foundation model is also crucial. A small model trained on high-quality, curated data can be more energy efficient and achieve the same results or better depending on your needs. IBM research has found that some models trained on specific and relevant data can perform on par with ones that are three to five times larger but perform faster and with less energy consumption.
- Locate Your Processing Thoughtfully
Often, a hybrid cloud approach can help companies lower energy use by giving them flexibility about where processing takes place. With a hybrid approach, computing may happen in the cloud at data centers nearest the needs. Other times for security, regulatory, or other purposes, computing may happen “on prem” — in physical servers owned by a company.
A hybrid approach can support sustainability in two ways. First, it can help co-locate your data next to your processing, which can minimize how far the data must travel and add up to real energy savings over time. Second, this can let you choose processing locations with access to renewable power.
- Use the Right Infrastructure
Once you’ve chosen an AI model, about 90% of its life will be spent in inferencing mode, where data is run through it to make a prediction or solve a task. Naturally, the majority of a model’s carbon footprint occurs here also, so organizations must invest time and capital in making data processing as sustainable as possible.
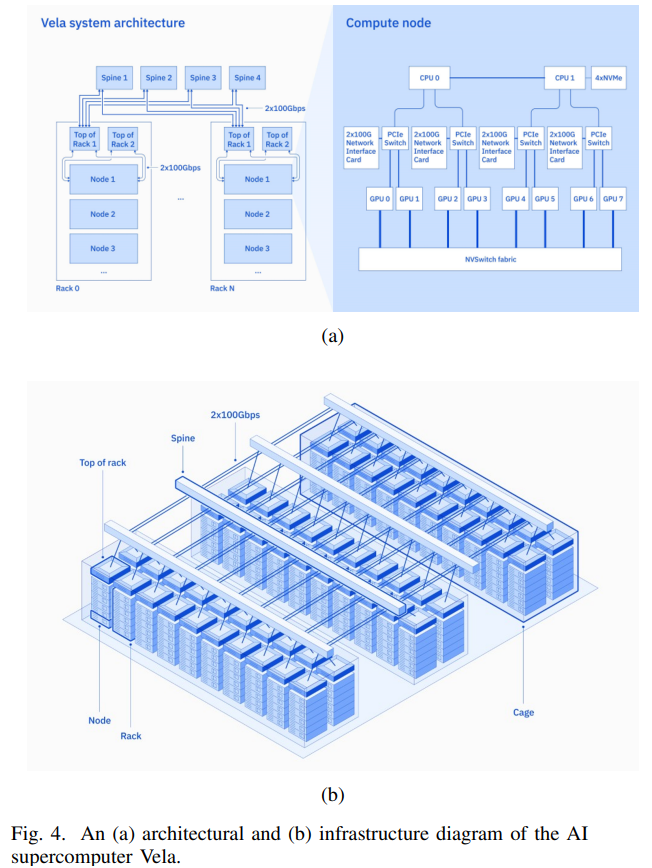
AI runs most efficiently on processors that support very specific types of math. Increasingly, we are seeing new processor prototypes designed from scratch to run and train deep learning models faster and more efficiently. In some cases, these chips have been shown to be 14 times more energy efficient.
- Go Open Source
Being open means more eyes on the code, more minds on the problems, and more hands on the solutions. For example, the open-source Kepler project — free and available to all — helps developers estimate the energy consumption of their code as they build it, allowing them to build code that achieves their goals without ignoring the energy tradeoffs that will impact long-term costs and emissions.
The Future of AI and Climate Change
The artificial intelligence boom has driven big tech share prices to fresh highs, but at the cost of the sector’s climate aspirations. Google admitted that the technology is threatening its environmental targets after revealing that data centers had helped increase its greenhouse gas emissions by 48% since 2019. Similarly, Microsoft has acknowledged that its 2030 net zero “moonshot” might not succeed owing to its AI strategy.
The largest and most expensive data centers in the AI sector are those used to train “frontier” AI, systems such as GPT-4 or Claude 3.5, which are more powerful and capable than any other. The leader in the field has changed over the years, but OpenAI is generally near the top, battling for position with Anthropic, maker of Claude, and Google’s Gemini.
The AI revolution poses a significant challenge to our climate goals, but it also offers solutions for greater sustainability. By making smart choices about AI models, locating processing thoughtfully, using the right infrastructure, and leveraging open-source solutions, companies can mitigate AI’s growing environmental footprint. Balancing the pursuit of AI advancements with sustainable practices is essential for a future where technology and the environment coexist harmoniously.